Pfizer Documents show Pfizer made its vaccine appear more effective than it was
Was it fraud? You tell me.
On May 24th, the anonymous Twitter account JikkyLeaks claimed that data contained inside the massive Pfizer Documents release shows their vaccine had close to zero efficacy even when it launched. There is some correcting of the raw data required to reach this conclusion, but the raw data alone hints at an efficacy of 53%. The data contradicts Pfizer’s published claims the vaccine was 95% effective, a claim still published on gov.uk domains. The original data and specifically the “95% efficacy” claim was key to getting the vaccines onto global markets around the world. If Pfizer reached this conclusion dishonourably, there could be sizable implications.
At least one other researcher has publicly reached the same results using data contained in the court-ordered Pfizer documents. I have already verified that the data exists, and using very simple public code, that the numbers add up to what Josh and Jikky claim. Understanding what they mean, and the potential implications is what I will now address here.


Not only has the specific claim been entirely ignored, which we might reasonably expect, but the existence of the documents themselves has also been entirely ignored. Against a backdrop of silence, this article is my attempt to unpack this data in an accessible way. To do that requires understanding how so many regulators around the world became dependent on ‘the word’ of Pfizer, a company with a proven track record of causing “false claims to be submitted to government health care programs”
‘The Rolling Review’
In November 2020, Pfizer started to make its successes with the vaccine known to the media. Towards the end of the month, they published a press release stating their vaccine was 95% effective. In the release, they said they planned “to submit within days to the FDA [and other regulators] for Emergency Use Authorisation.” With their guard down, the media didn’t hold these claims to account. Instead, and perhaps understandably, they choose to jump for joy. The regulators were pleased too; just two weeks after Pfizer’s press release, the UK regulator announced it had approved the Pfizer vaccine and that rollout would start within a week. Reporting the announcement, the British Medical Journal made note of something quite remarkable:
“No results from the trial have yet been published in a peer-reviewed journal.”
It was true. The Phase 3 trials of the vaccine were still ongoing, but the vaccine was now approved for use in the UK. The trials are still ongoing according to the registered protocol, which says they’re not scheduled to be complete until February 8, 2024. So how did the vaccine come to be approved before the full completion of Phase 3 trials? According to an FOI request, and a public article, the UK regulator addressed the question and said it had used a ‘rolling review’ process to approve the Pfizer vaccine. The review was done “as the packages of data become available from ongoing studies”.
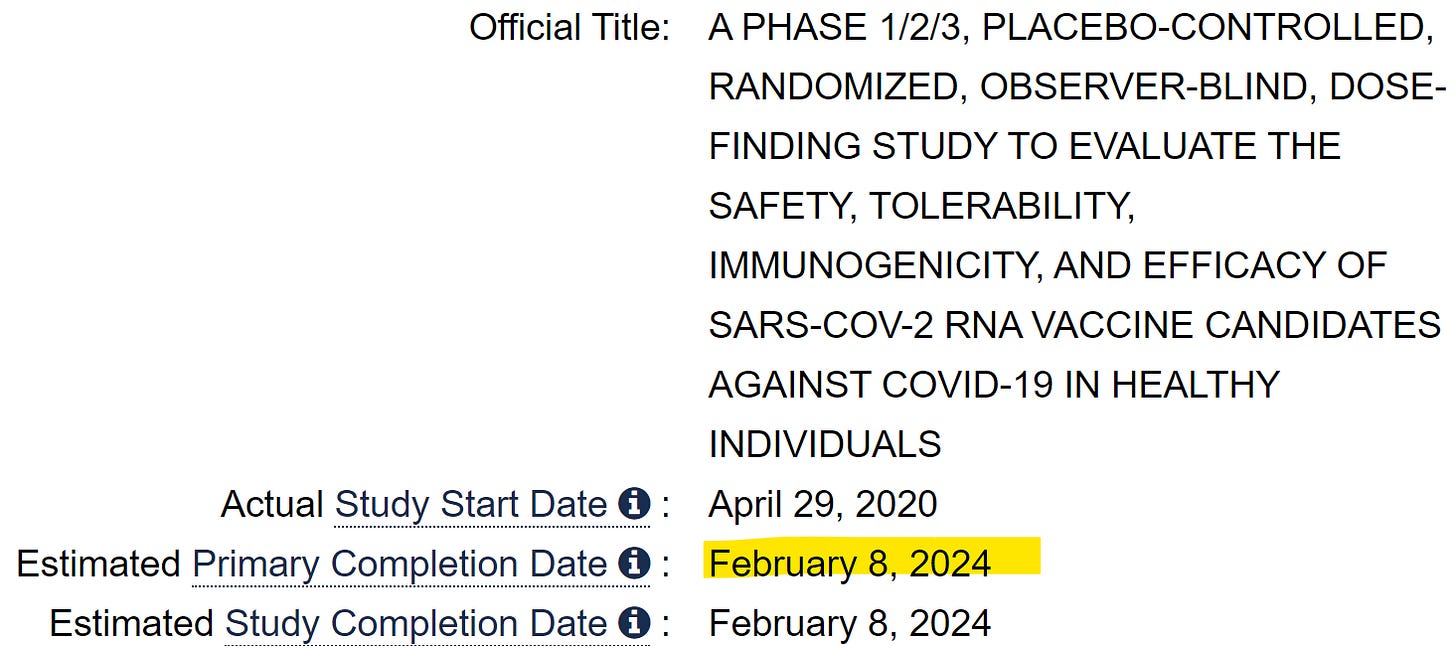
Whatever that regulatory process was, it wasn’t exactly public. To make matters worse, the trial had problems with impartiality and data sharing, which, two years later, is something that still frustrates Peter Doshi at the British Medical Journal.
“Pfizer’s pivotal covid vaccine trial was funded by the company and designed, run, analysed, and authored by Pfizer employees. The company and the contract research organisations that carried out the trial hold all the data. And Pfizer has indicated that it will not begin entertaining requests for trial data until May 2025”
Why wasn’t the data made public immediately? Describing the regulatory process, Pfizer CEO Albert Bourla said “Thirty of our people couldn’t sleep for five days… then the FDA would start. Then thirty FDA people would not sleep for five days.” He was triumphantly describing the process with Klaus Schwabb at the World Economic Forum in May of this year [22:28s]. It normally takes 10 years to develop and regulate a vaccine, but starting at the tail end of 2019, the whole process was done in 10 months. It’s worth noting, that had Pfizer wanted to slip an ineffective product past regulators, this might be a good way to do it. An exhausting back and forth of complicated and important trial data, all done in an impossibly short period, when everyone is desperate for a solution. What could possibly go wrong?
Whatever breakneck process was taking place at the FDA, things were even quicker at the UK regulator, because they approved the vaccine even sooner. They got it out of the door ten days earlier, in what looked like a political competition over who could approve the vaccine soonest. At the tail end of his administration, ruffled Trump officials demanded meetings with the regulator ‘to discuss timelines’ over the vaccine’s approval.
By skipping over the public phase which normally happens by publishing the studies in medical journals, oversight from the broader medical community had effectively been torched. It was now critically important that the information Pfizer gave to the regulators was accurate and gave a fair representation of the vaccine. So, was the data accurate? Did the incredible regulatory pace mean things were missed? And what exactly happened in that strange regulatory process?
A document called the “FDA Briefing Document“, prepared by Pfizer and published by the FDA on December 10th 2020 can give us some clues. The document is somewhere between a sales pitch and an executive summary of the trial data. The same information was presented by Pfizer to multiple regulators all over the world. It contained a critical piece of data that underpinned the “95% efficacy” line that Pfizer had been touting in the weeks before.
When June Raine and her team of UK regulators “robustly and thoroughly reviewed [Pfizer’s data] with great scientific rigour”, the part showing it to be 95% effective was of crucial importance. She said so herself, saying they had “looked at how the vaccine protects people from COVID-19”. So, Table 2, presented below, was mission-critical because it was the basis for the 95% efficacy claim. But there’s more to this claim than meets the eye. It marks the beginning of Pfizer misleading the public with their data.
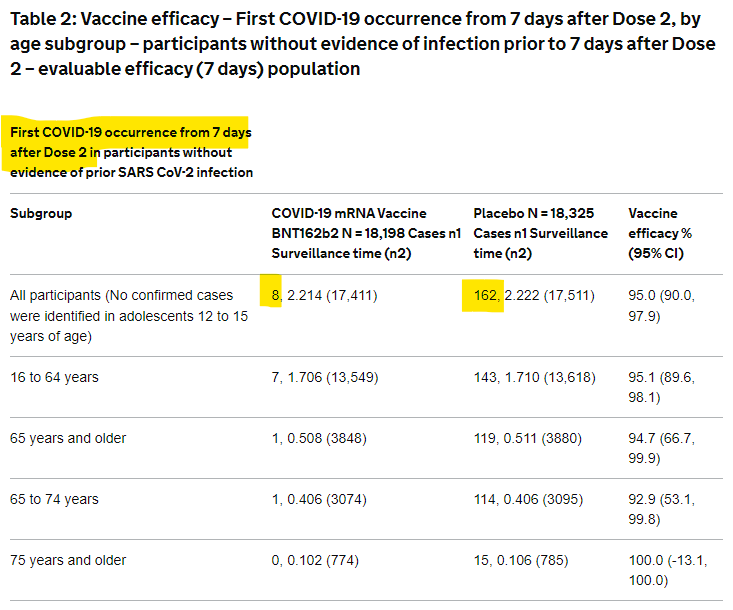
A Very Common Trick
Essentially, what the table shows is that of the trial participants taking the vaccine, only 8 of them tested positive for Covid-19 more than 7 days after their second dose. Of the trial participants taking the placebo, 162 people tested positive for Covid-19 within 7 days. The difference between those two outcomes is 154, which they use to calculate a 95% efficacy. How do they get there? The vaccine ‘reduced’ Covid-19 outcomes by 154, out of a total of 162, and since 154 is 95% 162, they say that’s “95% efficacy”. Does that feel correct? We’ll return to it shortly.
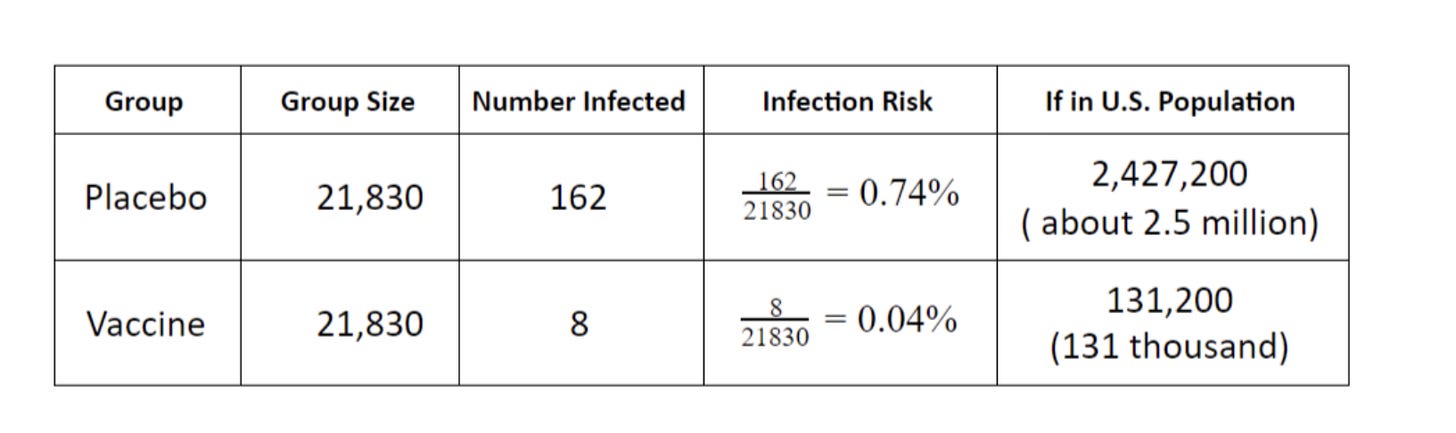
Nicely demonstrating how this data had been understood is an article from the New York Times which was published not long after Pfizer’s data had been released. As part of a revision guide for students, the article posted a table calculating how many people would be infected if we vaccinated everyone in America. Using the exact figures from Table 2, they calculated there would be just 131,200 infections across the entire United States. In reality, between June 2021 to June 2022, there were 52 million cases during a period that built towards 67% of the population being double vaccinated. So the discrepancy between the marketing and reality is massive, so what happened? Was the data wrong, or were they misleading people over what the data really means?
The first part of their trick is rooted in the gulf between what they hope is understood, and what the data really means. So we’re now going to unpack what the data means and see if that reality matches up to what we all think it means. This ‘doctoring of the data’ trickery is so rampant that its corrosive influence is rarely even acknowledged. It’s done so widely, at every stage of a drug’s development, that our understanding of these medical products is hopelessly and deliberately poisoned before these companies even start manipulating the underlying data. Before we can understand the ‘unacceptable’ form of manipulation that JikkyLeaks claims to have uncovered, we’ve first got to understand the manipulation that’s accepted as normal. Spoiler: what’s accepted as normal, is not normal.
Absolute or relative “efficacy”
Crucial to understanding the sleight of hand here is understanding what is meant by “efficacy”. I think it’s best imagined as the famous glass prism picture but in reverse. A world of diverse, complex, contradictory colours of data fire through a prism of glass, emerging out of the other side as “95% efficacy”.
The phrase has a ‘two-tier’ meaning; a public one designed to be simple and reductive, and a private one which implies clear qualifications by ‘those in the know’. This dual meaning makes the word incredibly useful because it placates two important but distinct groups of people; it can fool the public into believing the medicine is ‘effective’, whilst its implied qualifications keep ‘those in the know’ from being upset about its liberal use. Like a chameleon, it changes its colour depending on its context.
So how is it understood by the public? Well, Pfizer‘s “BNT162b2” product is understood by the public as a vaccine. It is described that way explicitly by the media, and it’s marketed that way by Pfizer themselves. Therefore, the public understands its ‘efficacy’ to mean its ability to create immunity to disease, in this case, Covid-19. So when Pfizer ran with their “95% effective” line, they know that what the public ‘hear’ is that the vaccine stops you from getting Covid-19 with 95% certainty. This is what Pfizer want the public, and even doctors, to understand.
But the medical world has much more precise discussions about what exactly the “95% efficacy” relates to. There are many factors that feature in “efficacy”, and they’re all concealed in that one singular word. Does it create immunity? Is it lasting immunity? How long does this ‘immunity’ last? Is it a relative risk reduction? An absolute risk reduction? What’s the confidence interval? Does it only reduce symptoms? By how much does it reduce the symptoms? How are we measuring a reduction in symptoms? Anywhere that social media permits, these questions are being debated right until this very moment.
The most critical component of “95% efficacy” is how it’s calculated. To get there, we’ve ignored the vast majority of the outcomes from the trial. Have a look again at Table 2. The overwhelming majority of people on the trial did not become sick with Covid-19, even if they didn’t receive the vaccination. If you look, you’ll see that out of 18,325 people in the placebo group, only 162 went on to get sick with Covid-19. That’s only about 0.88%. We can use this data to say the human immune system had a “99.2% efficacy” in stopping Covid-19? But that’s higher than the efficacy of the vaccine?! So what is going on?
All of this comes down to the fundamental difference between “relative risk” and “absolute risk”. In almost all cases when you hear how ‘effective’ a medicine is, you’re hearing how much it reduced relative risk. This only compares the difference in measured outcomes, it does not care about how minuscule those outcomes are. Let’s use an extreme example to demonstrate. Imagine someone came up with a shark deterrent, and to test its ability to deter sharks, they did a huge study; 10,000,000 people used the shark deterrent and 10,000,000 people didn’t. At the end of the study, there was a shark attack amongst the group of people not using the shark deterrent, and there were none in the group using it. Using the relative risk calculation, you can market the shark deterrent as 100% effective, because 1 is 100% more than 0. Is it fair? Maybe. But there’s always something concealed by the calculation; in 99.9999% of cases, no one was attacked by a shark anyway.
This is where absolute risk comes in. Baked into the number is your actual risk of the measured outcome in the first place. It helps to give us an immediate understanding of how at risk we are, and by what amount the intervention might help us. It also helps us better compare the risk-reward ratio if there are any side effects to the medical intervention.
In the paradigm we’re living in, absolute risk reduction is almost never communicated because it’s not good for business. So how do we calculate it? Let’s use the Covid-19 infection data from Pfizer’s Table 2. Left with no intervention at all, Pfizer’s data showed that your absolute risk of getting Covid-19 was about 0.88% because just 162 people out of 18,325 went on to test positive. For those in the vaccine group, the risk was about 0.04%, or 8 out of a total of 18,198. That means the vaccine reduces your absolute risk of developing Covid-19 by just 0.86%. We can even use this absolute risk reduction to work out how many people need to be vaccinated to stop one Covid-19 infection. It works out to 116 people. We need to vaccinate 116 people to stop one Covid-19 infection. Alternatively, we can say the vaccine is 0.86% effective.
Pharmaceutical trickery is baked into the very language we use to describe a clinical effect, so how can we reasonably expect to have a clear understanding of what’s going on? The difference between a 0.86% and 95% effective product is so vast that the public is left completely bamboozled, and this is the exact outcome that’s intended. The potential to mislead using relative risk calculations is large enough that the Association of the British Pharmaceutical Industry make it very clear that the absolute risks should always be communicated to the public. Here’s what they said.
Referring only to relative risk, especially with regard to risk reduction, can make a medicine appear more effective than it actually is. In order to assess the clinical impact of an outcome, the reader also needs to know the absolute risk involved. In that regard, relative risk should never be referred to without also referring to the absolute risk. Absolute risk can be referred to in isolation.
Had you ever heard the absolute risk figure before? Without it, you were unable to “assess the clinical impact of an outcome”. According to the ABPI, that you didn’t hear the absolute risk reduction figure means you were misled because by only communicating the relative risk reduction, Pfizer made the medicine “appear more effective than it is”.
There’s another word we can easily use to describe making something “appear more effective than it is”. Isn’t it a kind of fraud to misrepresent something for commercial gain? We were misled by a practice that’s so rampant that it’s considered normal, even though there are guidelines which explicitly say that both relative and absolute risks should be communicated. Without both numbers, we were flying blind. We were left without a proper understanding of what these vaccines could achieve. But this was just the first layer of the trickery…
The Fast Emerging Reality
In the manner in which it was understood, a “95% effective” vaccine would have ended the pandemic, but as we all know, it didn’t. For those paying close attention, it was clear that the vaccine didn’t work at anything close to the rate implied by Pfizer’s data, no matter which flavour of “effective” you wanted to use. A 95% effective vaccine just doesn’t tally with the reality we all lived through, but for several very human reasons, there’s still some emotional resistance to this. The resistance is understandable, because it’s exactly here, in the widely accepted understanding of “95% effective”, that the public were first conned. We were suddenly unable to make sense of what was happening because we had been misled about the efficacy of the vaccines.
To illustrate the vaccine’s real-world failure to demonstrate whatever “95% efficacy” means, I’ll offer a few examples. In Israel, infection rates broke records in the summer, long after the mass vaccination campaign had peaked. The graph below shows you that infections maxed out at 22,291 on the 8th of September, at a time when 60.2% of the entire population were fully vaccinated. If we consider only the eligible population, vaccination rates were running even higher at 80%. Israel was amongst the most vaccinated nations on earth at the time, and the vast majority of vaccines given there was the 95% effective Pfizer vaccine. Despite all of that, the spike in cases that followed vaccination was nearly triple the previous record of 8,450, set in the winter when vaccination rates were just 2.7%. Where a 95% effective vaccine fits into this picture is difficult to see.
What if we shift the definitions a bit? Instead of looking at the number of Covid-19 ‘cases’, we instead looked at hospitalisations? These are people, we presume, who were sick enough with Covid-19 that they had to go to the hospital. Even on hospitalisations, the data doesn’t nicely tally with a 95% reduction, as was touted both in the UK and the USA. In Israel, there was a very clear spike in hospitalisations after the vaccine rollout, and it actually exceeded the initial hospitalisation spike during the first wave. This happened despite a build-up in natural immunity from the first three waves of Covid-19. Does that fit with a 95% effective vaccine? Record cases and a relatively high number of hospitalisations? The graph below shows us many things, but it’s very difficult to square it with a 95% effective vaccine.
Again, we could look at the UK data and see a very similar pattern in hospitalisations. In the months that followed the vaccine rollout, there was another long and sustained wave of hospitalisations, but we expected something very different. After the approval of the Pfizer vaccine, England's Deputy Chief Medical Officer said the vaccine would “take out 99% of hospitalisations and deaths related to Covid 19." And yet, tens of thousands of hospitalisations followed, never returning to baseline even as I write this article 12 months later. How can we understand this in the context of “95% effective”?
One theory that often lurks in the subconscious to explain this incongruent reality is “imagine how bad it would have been if we didn’t have the vaccine”. It’s a folk theory of course, but because it plays some role in helping people understand the past two years, I think it’s worth shedding some light on.
The theory goes something like this: “the vaccine really was 95% effective and we were witnessing Covid-19 rip through society at 5% of its full potential.” It’s a line of reasoning hinted at by many public figures on Twitter. If we defanged Covid-19 to the tune of 95%, it means we only saw 5% of what was coming our way. Under such a “95% reduced” scenario, we avoided 20x higher daily hospitalisations. We avoided a peak of 400,000 people hospitalised in a single day, that’s 3x the total of all the NHS beds in the United Kingdom. It means we avoided 3.5 million deaths, roughly 5% of the entire UK population. It means the vaccine prevented an armageddon of sorts.
But we can test this theory by looking at countries with low vaccine uptake for evidence of a scenario as awful as this. As of writing, Nigeria is only 9.7% fully vaccinated, a country of 200 million people, but no such armageddon has so far befallen them.
Other theories might explain what we saw; a vaccine that wasn’t working as claimed, ‘cases’ not being truly reflective of ‘Covid-19 cases’, hospitalised patients being overcounted, or a combination of all of the above. None of these theories were allowed to be discussed because of very tightly controlled public health messaging. As I previously reported, you were either ‘on brand’ for public health messaging, or you were silenced. These fairly asinine explanations for the fast-emerging reality were not ‘on brand’, so they were never allowed to filter into the public consciousness. Speculation was banished to private conversations with friends or admirably brave podcasters.
From a narrative perspective, we were in the Twilight Zone. Perhaps if the public had been told the vaccine had an efficacy of 0.86%, things might have made much more sense.
The Narrative Fudge
Having repeated Pfizer’s claims that the vaccine was between 90% and 95% effective, how could regulators accommodate the fast-emerging reality of record covid cases? It required a fudge because the public were expecting something very different. First, we were told the vaccines were preventing transmission, but at the same time, we were beginning to hear they were not. It’s beyond the scope of this article to detail the censorship and finger-wagging that followed as scientists attempted to have a rational discussion about this. Needless to say, regulators eventually admitted defeat and by late July, they quietly announced that the vaccine didn’t stop transmission.
Their wording is worth paying very close attention to. “Unlike with other variants,” said the CDC, “vaccinated people infected with Delta can transmit the virus”. Read it again.
It was a huge retreat, but contained within it was the seed of a vital story which would provide cover for the fast-emerging reality. With cases at record highs, the narrative underpinning the mass-vaccination program shifted focus. Officially, it was now about the vaccine’s ability to “reduce hospitalisation and death.” By refocusing on a different measure of success, the room was created to accommodate the record case rates. It was a narrative fudge, and it started to appear in the aftermath of the Delta wave. It sought to rationalise high cases with a story that came to life via the CDC’s quiet retreat. The narrative was something like this, “The vaccine was 95% effective against the previous variant, but this is a different strain… Delta… there are mutations… we need to vaccinate more.”
Perhaps it was true.
There was, after all, a brand new strain of Coronavirus ripping through society. Its at least plausible that Delta alone explained the unprecedented rise in cases. So let’s take that narrative at its word. The “95% efficacy” of the vaccine waned because of new variants. More or less, this is the perspective that’s held in many mainstream circles to this day. The perspective is malleable enough to accommodate the important truth: at one time the vaccines did have “95% efficacy”. That fact is crucial because it’s what Pfizer told the regulators. It’s what the CDC said about the “other variants”. It’s a fact still published on government websites. It’s a fact critical to the emergency vaccination campaign and it was the backbone of the overwhelming health messaging we were subjected to.
Leaving aside the trickery of absolute vs relative risk reductions, what if there was another explanation for the high case rates after vaccination? What if Pfizer’s data never showed the vaccines to be effective in reducing Covid-19 at all? What if that data, which had been given to regulators all over the world, had been selectively edited in such a way as to make the vaccine appear more effective than it was? Understandably, such a reality would be unfathomable for many people. We’d be looking at one of the largest medical frauds in history, so you’ll excuse me if I tread carefully.
If we look dispassionately, a vaccine with an overstated efficacy might go some way toward explaining the record case rates which appeared right after vaccine rollout. It might explain how we saw the exact opposite of what we’d expect from a 95% effective vaccine. Governments, content that they’d vaccinated society, lifted restrictions and the public started to mingle thinking they were protected, but with a faulty vaccine, cases exploded. It’s… plausible, but does the data support it?
Enter The Pfizer Documents
Cast your thoughts back to Peter Doshi, editor of the British Medical Journal. Frustrated at the lack of transparency over the Pfizer trial, in January 2021 he said, “We must have raw data, now. …Pfizer has indicated that it will not begin entertaining requests for trial data until May 2025.” In seeking to expedite that process so that researchers could have access to that data “now”, The Guardian chimed in and described the process as “a legal blitz to sow disinformation.”
Quite.
Whilst Doshi’s demands for data were admirable, he may not have been aware that two weeks earlier, those ‘disinformation’ campaigners had already announced their victory. Instead of a schedule allowing Pfizer’s trial data to be published over 75 years as both Pfizer and the FDA had wanted, a judge ordered Pfizer and the FDA to produce all of the data in a matter of months. Abiding by the court ruling, Pfizer has kept to the schedule and has been sending the data to Public Health and Medical Professionals for Transparency, who host it on their website. It was a huge victory.
It will come as no surprise to you that the data has been almost entirely ignored. Worse still, it’s bordering on the incomprehensible because of its terrible ‘picture of paper’ formatting. Progress on deciphering it has been steady, and so we now have access to data that we didn’t have before. But even if we can now see it, what on earth does it say?
Enter JikkyLeaks. Our anonymous mouse.
JikkyLeaks and a growing number of public-facing scientists think that new data from the Pfizer Documents might support a ‘no efficacy’ theory. By that, they mean that the vaccine doesn’t work at all. It’s certainly a radical proposal, so… what does this new data show?
To understand JikkyLeaks’ claim that the Pfizer Documents contradict the “95% efficacy” claim, we must understand exactly how Pfizer calculated it in the first place. It requires getting into that ‘colourful rainbow’ of data being fired into the prism. So let’s get started, don’t worry, I’ll make this fun…
When confirming who did and didn’t get Covid-19 on the vaccine trial, “cases were determined by RT PCR and at least 1 symptom”. So, patients needed to be PCR positive and have one symptom to be a confirmed ‘Covid-19’ case. According to the protocol, suspected Covid-19 cases were swabbed by a clinician, and the sample was then “tested at a central laboratory.” Quite why the swabs weren’t tested right there in Argentina is anyone’s guess. In any case, this is how we got our 162 placebo cases, and 8 vaccinated cases.

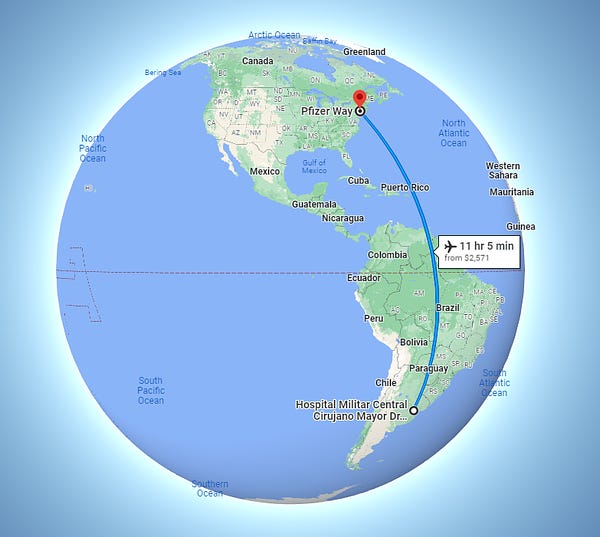
Was it a good way to count them? It’s certainly not perfect. The PCR test is known to be faulty in determining cases. Famously, PCR’s eccentric inventor said the results of the PCR reaction “make a whole lot of something out of something. That’s what it is. It doesn’t tell you that you’re sick”. Without getting bogged down in a debate about PCR tests, there’s at least one thing worth knowing about them. They amplify incredibly tiny amounts of genetic material to a level where we can measure it. They’re so sensitive that even waving a PCR swab through the air of a room where an infectious Covid-19 patient was sitting can be enough to give a positive result. But inversely, they can only detect virus particles if they’re sitting in the back of your throat where the clinician swabs for them. So the tests are not without their problems.
The PCR test isn’t sufficiently solid enough on its own to confirm a case, that’s why Pfizer combined it with an additional ‘soft endpoint’ of patients expressing “at least 1 symptom”. But this is a subjective endpoint because it requires clinician judgement. Did someone’s shortness of breath slightly increase or not? Did their existing cough deteriorate slightly? A human interpretation is subject to human bias.
A positive PCR test along with the expression of a symptom is sufficiently malleable that if a clinician was suitably motivated to, case rates could be fudged. If falsifying records during a pivotal vaccine trial sounds implausible to you, have a look at what Brook Jackson reported to both the British Medical Journal and myself here at The Digger. There definitely were problems in the Pfizer trial, and that’s putting it lightly. Brook Jackson is a credible whistleblower, vetted by the BMJ, and she maintains that trial managers were forging and falsifying data on case report forms. In one case she alleges they changed a patient’s record of symptoms to be “Covid-19 related” when it had originally not been. The BMJ were careful and called the problems “data integrity issues”. I think they’re better described as fraud.
Noting the evidence we already have of forging data, and given what we saw in the real world after the vaccine rollout, is there any new data inside the Pfizer Documents which might help us to check Pfizer’s homework? To test the “95% effective” claim would require another dataset to tell us how many people on the trial became infected. Ideally, the dataset would be at least as sure as “PCR positive and 1 symptom”. If such a dataset existed, it should show us a similar pattern as was reported in the official data. We should see something close to a 95% reduction in cases amongst the vaccinated over the placebo group. This would be a reasonable ‘check’ that Pfizer’s count of infected trial participants was accurate.
Well, it turns out that there is another way that we can test for prior infection to Covid-19, and the data is sitting there inside the recently released Pfizer Documents.
Pfizer tested for N (nucleocapsid) antibodies when trial participants came to the clinic as part of their regular checks. The test measures whether your body has developed antibodies to the N protein of the SARS-Cov-2 virus. A positive anti-N test “confirms previous infection” according to the UK Government. Crucially, you can’t get anti-N antibodies from the vaccine itself, you can only get them from a meaningful exposure to the SARS-Cov-2 virus. So detecting them in the trial participants’ blood means those patients were infected with SARS-Cov-2.
This is where JikkyLeaks spotted an opportunity. It’s possible to count up the number of trial participants who were negative at visit 1 and then positive at visit 3. These are patients who got infected with SARS-Cov-2 during the study period in such a way that they developed the N antibodies. Counting ‘positive cases’ this way, gives us 160 cases in the placebo group, but 75 in the vaccinated! That’s a huge discrepancy since we should be expecting something closer to the 8 figure reported by Pfizer in Table 2!
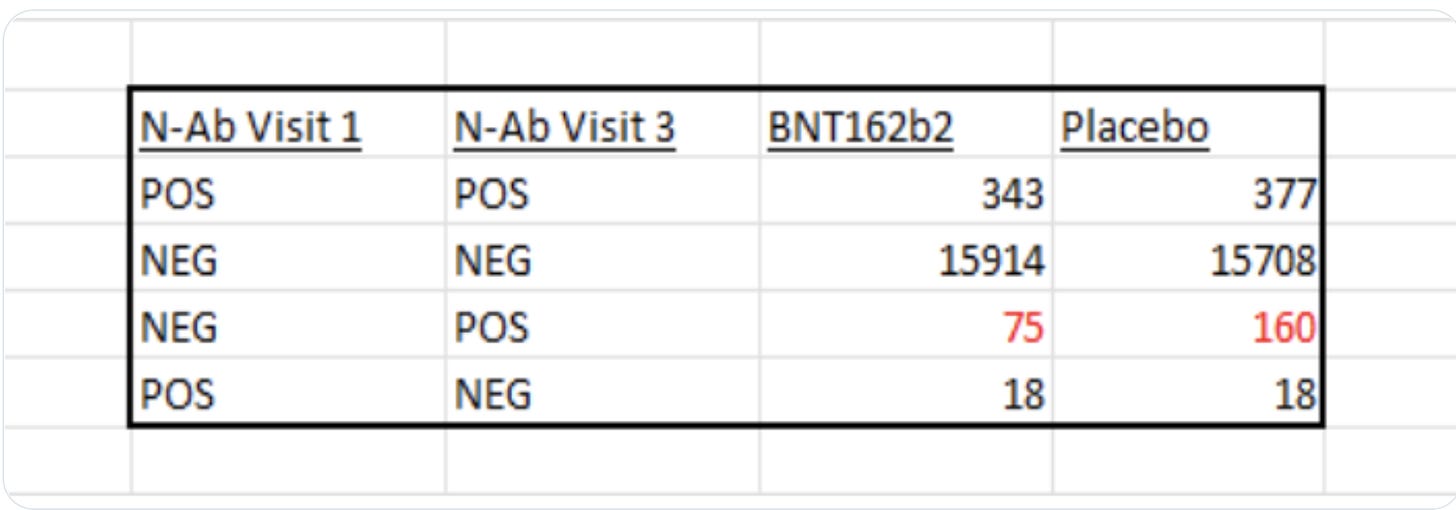
There are a couple of things that stand out with these numbers. Firstly, the sense checks on other combinations of patients who went from “Positive” to “Negative”, or “Positive” to “Positive” come back as we’d expect - they’re broadly the same. Secondly, 160 positive patients in the placebo group is very close to the original 162 presented in the data given to the regulators. So two different sense checks hint that our new dataset lines up nicely. In the placebo group, we’re finding close to what we’d expect to find.
But not all of it lines up as you’d expect.
In the vaccinated group, we’re seeing 75 patients who went on to be infected with SARS-Cov-2, which is 9x higher than the 8 Pfizer presented in their original data. It’s a big difference that’s not easily explained. If we were to only focus on these numbers, they demonstrate a (relative risk) efficacy of just 53%, which is a far cry from Pfizer’s claimed efficacy of 95%. The numbers are accurate, you can use my codebase to check them for yourself. Before we go any further, it appears that Pfizer’s data contradicts itself; their “95% efficacy” halves when you measure infections via anti-N results. But the discrepancy only gets worse from here.
What JikkyLeaks and Josh Guetzkow are keen to highlight, is that a recent study shows that the mRNA vaccinated express N antibodies at a lower rate than the unvaccinated. In this study of 1789 patients exposed to SARS-Cov-2, N-antibodies were found in only 40% of vaccinated patients compared with 93% of unvaccinated patients. It means that if we’re looking to see all the ‘positive’ cases by counting for N-antibodies, we’re only seeing 40% of all the cases. To get closer to the ‘true’ number, we would have to correct it upwards, to account for N-Antibodies only appearing in 40% of the positive cases.
The adjustment works out to 2.3x what we already calculated, and when we apply that correction, this is what we get. There’s now no meaningful difference between vaccinated and unvaccinated. We’re now at zero efficacy using Pfizer’s measure of anti-N positive tests and one simple correction.
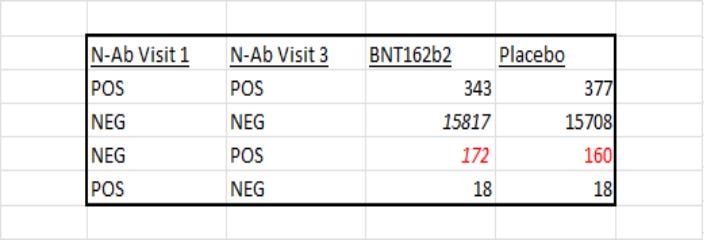
The implications of this being correct are substantial, so to publish I have to be sure there’s enough here to warrant our attention. We’re talking about Pfizer’s data contradicting their very public claims about the vaccine’s efficacy. Something that, as we’ve seen, is plausible based on what we saw after the vaccine rollout. And it’s plausible given Pfizer’s track record and the ongoing Brook Jackson whistleblower case.
Thinking critically about the data, there’s a line of reasoning that had me a little concerned over whether these results are even meaningful. These numbers alone do not show us something crucial; symptomatic infection. The patients here went on to be exposed to SARS-Cov-2 and develop N-Antibodies, but so what? What matters is whether they got sick or not, and it’s not readily apparent from these numbers alone that they did fall sick. That’s why Pfizer measured if PCR-positive patients also developed at least one symptom.
So do these results even matter? The vaccine can’t stop you from being exposed to a virus. So perhaps the vaccinated group in this dataset were exposed to SARS-Cov-2, and after exposure, they developed N-antibodies without even noticing. They might have never fallen sick with the illness of Covid-19, and if these patients didn’t actually fall sick, that would be evidence that the vaccine was doing its job. This question needs answering if these results are to mean anything at all. Is there a link between a positive anti-N test and people ‘being sick’ with Covid-19?
Well, I found that there is a link.
The study we used to inform our ‘correction’ of this exact data looked very closely at the prevalence of N-Antibodies. The authors said, “higher SARS-CoV-2 viral copies at diagnosis was associated with a higher likelihood of anti-N Ab seropositivity”. Ergo, the sicker you were, the more likely you were to develop these antibodies. The relationship is very clear on the graph below which is taken from the study. It shows the viral load on the X-axis and the probability of developing N antibodies on the Y. Put simply, vaccinated patients had to have higher viral loads to develop these antibodies. The graph shows that the presence of N antibodies is linked to a patient’s viral load, a proxy of their relative ‘sickness’.
It’s not the only study that demonstrates the link, there’s more evidence here, here and here. There’s this study in nature that showed that “asymptomatic individuals had a weaker immune response to SARS-CoV-2 infection", suggesting that it’s mostly those who were symptomatic that go on to create a detectable immune response. The large-scale Zoe Covid Study in the UK also found a link, “people who had a greater number of symptoms while they were ill… were more likely to have gained antibodies against the virus.” This study even showed that anti-N tests can pick up positive Covid-19 cases where the PCR test misses them because the PCR test was missing cases where the virus was sitting lower down in the throat. This could help to explain why we’ve found more Covid-19 cases than Pfizer reported because the anti-N data is picking up cases that the PCR test missed.
To put it simply, there’s a very credible link between testing positive for anti-N and having had symptomatic Covid-19. The extent and exact detail of that relationship will no doubt be debated, but the relationship is clear enough that these findings are meaningful. So what does it all mean?
More vaccinated patients got sick with Covid-19 on the Pfizer trial than Pfizer claimed in their ‘Briefing Document’ which was used all around the world. It raises serious questions, why didn’t they include this data in their Briefing Document? In the entire 53-page summary, antibodies are mentioned just once. This data in particular is nowhere to be found. Why?
It also raises questions for the regulators. Did they have access to this data, and was it checked before the approval? When June Raine and her team “robustly and thoroughly reviewed” the data, did they see this antibody data which contradicts Pfizer’s claims in the briefing document and press campaign? If they robustly and thoroughly reviewed the data, perhaps they saw this anti-N data and have an explanation for why it contradicts the PCR data in such a sizable manner? Perhaps they’ve looked at it and are satisfied there’s nothing to it? If they have such an explanation, we should welcome the chance to hear it.
Or did they miss it because Pfizer never presented it to them? It certainly wasn’t contained in the ‘Briefing Document’ which appeared right at the moment Pfizer got their vaccine approved. What exactly did the regulators have access to when they gave the nod on this vaccine for which the efficacy now appears to be a mirage?
There’s a very big difference between the trickery that goes on in how numbers are represented (relative vs absolute risk) and manipulating the numbers directly. The industry and the media will tolerate the former, even to the detriment of public health, but the latter is much more serious. The biggest question which must be answered is why the discrepancy is there at all. I’m sure we’ll hear good-faith explanations, and I’d welcome those. But given the testimony of Brook Jackson, and Pfizer’s checkered history of manipulating regulators, we also must be prepared to accept that the convenient difference between these two datasets is deliberate.
One dataset was published extensively in the press, and the other dataset they tried to bury via the courts. An obvious question appears from such a scenario; why?
If you liked this article, please consider becoming a paid subscriber. My incredible paid subscribers are the reason I can create this work, and I have plans to create even more. Also, this article has no audience at all without your shares, so please do share it with people who might like it.
This is a brilliant exposition & very clearly written. Thanks so much!!
Btw, an early study from Italy (Systemic and mucosal antibody secretion specific to SARS-CoV-2 during mild versus severe COVID-19; https://www.biorxiv.org/content/10.1101/2020.05.21.108308v1 ) reported that:
“Interestingly, we were able to detect S protein-specific IgA in the mucosal samples of several subjects in the absence of seropositivity. Additionally, a few other individuals also had detectable S protein-specific IgG in their nasal fluids despite being IgG seronegative”
and
“Interestingly, in 15–20% of S protein-seronegative individuals, we were able to detect S protein-specific IgA antibodies at several mucosal sites. Furthermore, mucosal S protein-specific IgA levels inversely correlated with patient age, suggesting increased mucosal antibody responses in younger SARS-CoV-2-exposed individuals”.
Considering that the trial participants were heavily tilted in favor of younger ppl, well.....
I wonder what might turn up if there was mucosal antibody surveillance on trial participants, which could capture the more mild cases that didn't seroconvert. If for instance in the placebo there is higher proportion/incidence of mucosal Abs w/o humoral Abs, that would imply that *severity* was higher in the vax arm.
👆 THIS 👆 Is why I'm a paid subscriber!